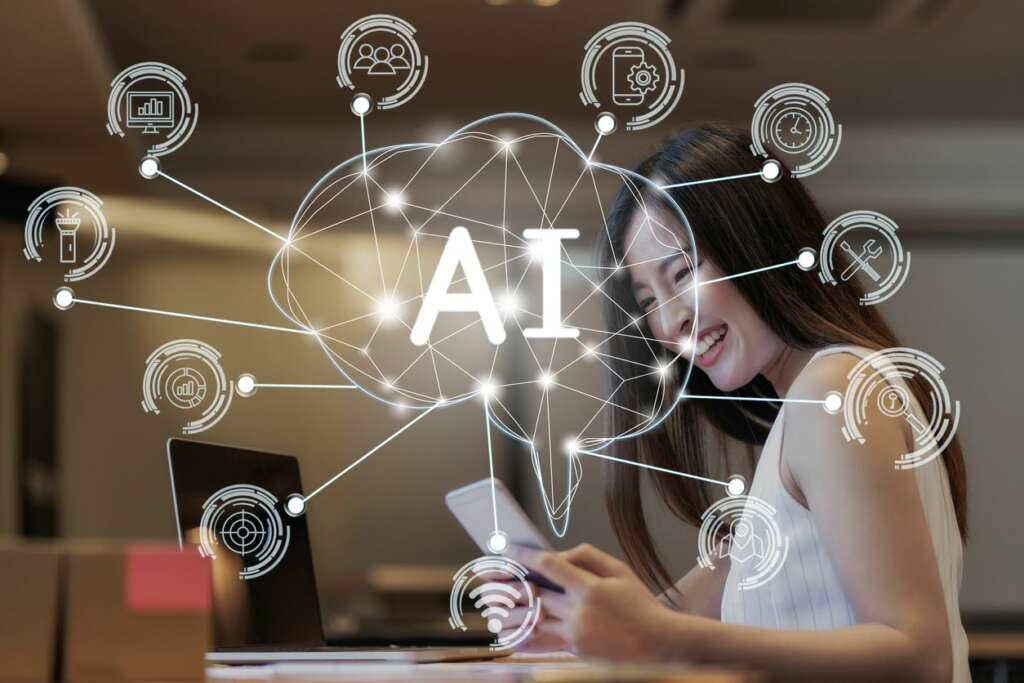
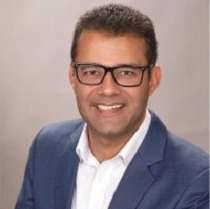
By Jasneet Kohli, Global Head/VP of Solution Consulting, antuit.ai
The traditional retail and consumer packaged goods (CPG) playbook doesn’t work anymore, and companies are using new data sources to gain an edge. But this data capturing goes beyond the need to capture data for data’s sake – intelligent systems use the data to drive inventory decisions. Wine companies can use weather data to plan their shipments to avoid excessive heat, and health companies can utilise pollen data to place allergy medicine in suitable locations. And that’s just the start.
The past few years have been chaotic, to say the least, with retail and CPG companies experiencing both inventory shortages and gluts. The IHL Group recently published a study that put the total cost of overages and shortages at nearly $2 trillion – up 12.7% since 2020 – with$1.235 trillion of this cost due to out-of-stocks and $758 billion due to overstocks.
When cash is locked up in inventory, companies don’t have the freedom or agility to make the necessary investments. And the cost of being understocked is not limited to lost sales. According to various studies, the primary reason consumers try another brand is availability. That may not be a surprise. It’s probably not a shock that empty shelves are the primary reason consumers leave a store without purchasing, either.
This is a call to action – and specifically a call for change – from the first mile in the retail value chain through the last. Retailers and CPG companies that implement new technology solutions without changing how they’re using technology aren’t solving anything.
A Trifecta of Innovation
There’s no doubt technology is key to solving tomorrow’s problems. It has made a significant impact in the recent past. Due to American companies’ technology investments, labour productivity grew by 2.2% annually between 2020 and 2021, up from a 0.9% average annual growth from 2011 to 2019. And in IHL Group’s beforementioned report, “Better systems and processes have provided $23.9 billion in sales improvement in the last two years alone.” Similar things are being seen across businesses in the UK including the manufacturing sector.
Cloud computing increases processing power while lowering costs. SaaS subscriptions force software providers to be more accountable for their promises. And artificial intelligence (AI) solutions decipher data at escalating speed, scale, and sophistication. It’s a trifecta of innovation.
Despite the excitement, AI technologies alone are not a magic bullet. After all, many AI projects fail to deliver expected results, and transformative results require human interaction with the AI system. Business users don’t have to know the details behind how the AI works, but they should conceptually understand what it’s doing and how to use it.
Transformation Through Augmentation
The biggest challenge that CPG companies face today is anticipating consumer demand. Surveys of UK supply leaders cite a cocktail of hurdles. According one survey, over seven in ten (72%) UK businesses are currently holding items, including £3bn of food and drink in their warehouses awaiting completion because raw materials, ingredients or component parts have not yet been delivered from suppliers.
Supply chain issues compounded by shifting buying behaviour have inventory whipsawing from shortages to overages. Using AI to augment systems is a viable solution. System augmentation is not new, but before, it added new features and capabilities onto a core enterprise resource planning solution when that functionality wasn’t there. System augmentation for AI adds intelligence to get the number right. Let’s take a typical situation.
Demand planning is executed through a system that provides multiple data views, drives down into details, combines information across channels, and allows you to adjust the plan. But suppose the underlying forecast isn’t correct or doesn’t use the proper demand drivers. Does the demand planner bump it up or reduce it based on their personal knowledge?
If the answer is yes to either, the value is diminished as people will always have biases. In the next step, sales and marketing teams add their projections to establish a consensus. But is that new number accurate? Probably not. And how quickly can you adjust this plan according to this process? Yearly, biannually, quarterly? When is it too late?
The benefits of augmenting your solutions with AI are maximising your current investments, increasing innovation speed and doing so at a lower cost.
Suppose you have spent millions on a replenishment system that functions well, but you are unsatisfied with the results it’s providing you. The problem is that system is already integrated with your warehouse, ordering, and inventory management systems. If you replace the replenishment system, those other interfaces will need to be reworked – for no added benefit, by the way.
You’re also going to have to retest your processes and retrain your staff. Not to mention all the new issues that will always appear when implementing software. All these refinements require specialists. That means you’re also pulling your people from their day jobs to support the specialists, however long it takes to get to a minimally viable product state.
Conversely, if the core issue is that you are struggling to keep up with consumer demand shifts, then the issue may not be the system itself but the forecasting and intelligence behind it. If you could infuse more intelligence into the system, you can mitigate your largest problem and drive additional value out of your current systems without having to go through any of the more resource-intense refinements I previously described.
Does this mean you never need to replace that system? No. A new replenishment system provides you a fix to your most important issue faster and cheaper than trying most other options. Just make sure you choose one that enables you to keep the intelligence extracted and stored by your previous system while improving the execution portion of demand planning.
This system augmentation approach has already been proven. David Simchi-Levi, a professor and head of the MIT Data Science Lab and Kris Timmermans, a senior managing director at Accenture, showcase how to spend less and accomplish more in their Harvard Business Review article. The piece states that by leveraging “a consensus forecast with a unified view of demand” as the foundational initiative, they can lower the transformation costs from tens of millions and a 35-year timeframe to a few million and a timeframe of 12-24 months.
The Familiar Tug of Hope
Despite the “whack-a-mole” atmosphere with new issues rising, and others seeming to disappear only to suddenly appear again, we’ve continued to see improvements through technological advancements and human ingenuity. Retail and CPG companies welcome data, along with AI, machine learning, and innovative ideas to gain that edge. So, as we get into the new year many of us feel that familiar tug of hope for better results and methods in the workplace, retail stores and other places where our personal and professional lives intersect.