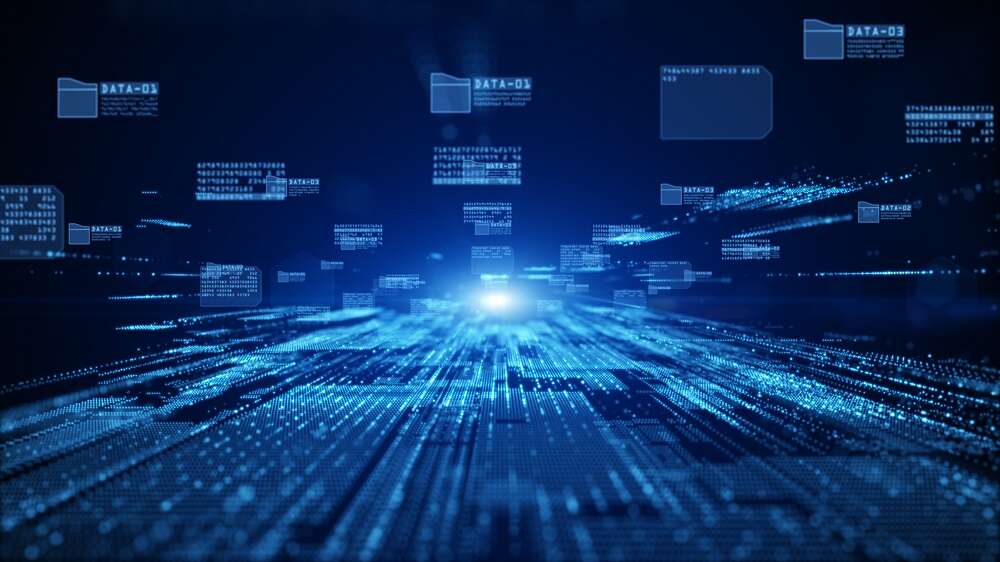
By: Douglas Greenwell, Chief Strategy Officer, Duco
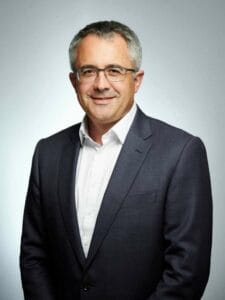
Douglas Greenwell, Chief Strategy Officer, Duco
Traditional methods of data quality management in financial services organisations are often still very manually intensive. Most organisations recognise this problem and are increasingly looking to replace these legacy systems and manual ways of working with new processes and technology because the cost of not doing so will very soon become too much to bear.
Good quality data is the cornerstone of compliance and empowers intelligent business decision making using the latest insights. If the quality of data is low, organisations are likely to lose revenue, miss important opportunities for the business (such over looking a customer need that their competitors may capitalise upon) or potentially even become non-compliant.
Functions such as data reconciliation which broadly ensures the accuracy and alignment of critical company data between systems, is particularly essential for data quality. However, it has traditionally cost financial firms heavily due to the effort involved in maintaining legacy systems and the number of employees needed to conduct reconciliation manually. And with these armies of people comes significantly increased risk of human error which exacerbates the issue.
To resolve such challenges financial services organisations should be stepping up efforts to combat data quality issues through mature data reconciliation practices and replacing inefficient and error-prone processes with automated systems to accurately reconcile data. But are they?
To find out, we commissioned a survey of 300 heads of global reconciliation utilities, chief operating officers, heads of financial control and heads of finance transformation working in large financial services organisations.
(Over)reliance on manual systems
Our survey found that nearly one in five financial services organisations rely totally on manual processes, with 87% having between 11 and 40 manual controls or spreadsheets for reconciliation tasks.
The slow and error-prone manual processes are having a significant impact on the quality of data with 42% struggling with poor data quality and data integrity within their organisation. Furthermore, 36% say they ‘hate’ the way they reconcile data, while 41% say data reconciliation is stressful and something they lose sleep over.
Despite the difficulties related to data quality and manual processes many organisations are reluctant to initiate change, particularly due to the associated cost and disruption. As shown by the survey, 44% believe that reconciliation without manual processes would be too challenging due to the different types and sources of data they are dealing with. And more than two fifths (42%) are of the opinion that the benefits of data automation are not worth the risk of disrupting the business.
Drivers of change
To gain an even deeper contextual understanding of the current state of play, let’s explore the ‘reconciliation maturity model’ which outlines four stages of maturity as organisations move from manual to automated reconciliation systems.
The first stage — the Manual stage — describes an organisation which carries out all reconciliations manually, usually with the help of spreadsheets. The second, Hybrid stage, means that there are reconciliation systems in place for all specific data types. The Improving stage describes a position where one automated intelligent system is used to reconcile all data. Finally, the fourth stage — the Automated stage — is one where all reconciliations are consolidated via automated systems.
When reviewing our survey data, from the perspective of the reconciliation maturity model, there are reasons for optimism. More than half of financial services organisations actually fall into categories 3 and 4 on the maturity model, with 31% operating a fully automated reconciliation system.
Just under half (45%) of financial services organisations are also actively investing in ways to improve data reconciliation and precision to reduce costs, get ahead of the competition and reduce the risk of regulatory non-compliance and associated fines.
Other key drivers to improve reconciliation include reducing the risk of fraud, and improving operational agility and resilience. While agility and fraud are big drivers, often the biggest business case for change is simply cost control.
Data automation also presents an opportunity for technology rationalisation by reducing the Total Cost of Ownership related to data normalisation, data prep and the infrastructure needed for hosting, running and upgrades.
Towards self-optimising reconciliation
Enabling technology for automated reconciliation has vastly improved over the past few years. Modern Intelligent Data Automation (IDA)approaches use an ecosystem of no-code, cloud-based tools to automate and control all financial, operational and commercial data across an organisation. By using fully customisable, low-cost solutions that can sit alongside or on top of legacy systems, an IDA approach is the key to not only successfully managing data, but to unlocking the full benefits of that data for the business.
Our survey indicates that within just a few years, we will see financial organisations move further up the reconciliation maturity model with the help of IDA and machine learning.
In fact, machine learning has already gained significant traction in the sector. One third of financial services organisations are using machine learning for some of their data, and 47% say they play a role in how they reconcile most of their data. Looking ahead, 42% of respondents say they will investigate the use of machine learning for the purposes of intelligent data automation.
Importantly, IDA is not a goal in itself, but rather presents a path to self-optimising reconciliation where the process automatically improves over time, saving significant amounts of money and time. By employing this over-arching, self-optimised level of automation, the IDA approach enables businesses to get a detailed view of data across the enterprise, no matter what that data is and where it resides.
With this level of insight, financial services organisations can better understand the performance of their operations, uncover and address weaknesses and identify new opportunities, all of which drives greater efficiency and agility across the organisation.
At the beginning, I highlighted how good quality data underpins intelligent decision making and compliance. Data quality in turn relies on sound data reconciliation practices and a single view of data across the organisation. This is the key which IDA and self-optimising reconciliation is set to unlock, bringing new efficiencies and insight, while safeguarding compliance.