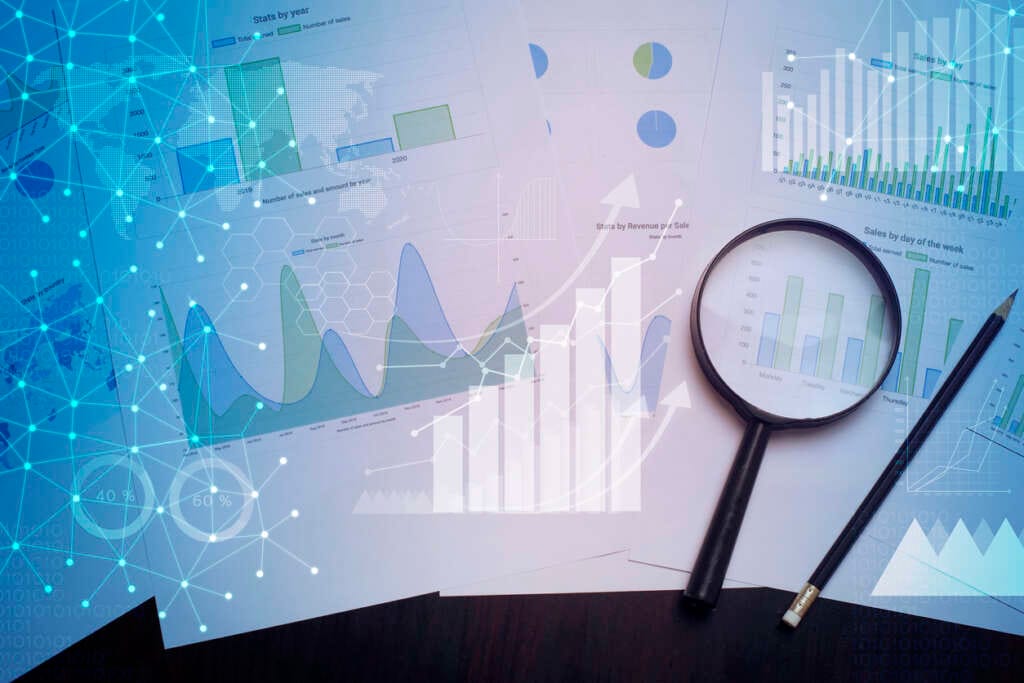
Embracing AI in the world of data analytics
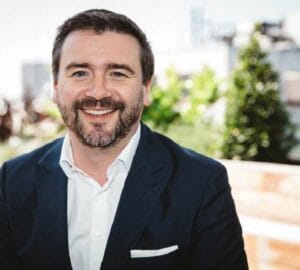
By James Fisher, Chief Strategy Officer, Qlik
The creation of the internet led to some uncertainty about how it could be used and the impact it might have on our daily lives. Some parallels can be identified between that and the current rise in generative AI. The uncertainty of how this new technology will work cohesively in our work, education and personal lives has created a sense of fear, as we don’t yet know how to interact with this new technology or even its full capabilities.
Data analytics has been particularly revolutionised by AI. For example, generative AI needs data to function, its systems have been trained to learn patterns, relationships and structures within data. Ultimately, this serves as the basis for the model to generate new and meaningful conclusions.
For organisations, having their ‘data house’ organised is where it begins. A recent article from McKinsey highlights the importance of companies assessing their data architecture in order to scale up the use of AI to provide greater data insights.
Four areas of data should be controlled to ensure a successful approach when combining generative AI with analytics, and this includes the following:
Data integration
Bringing data together from a variety of sources is essential to using AI. Today, data is generated by a huge amount of organisations and sources, such as mainframes, SAP, files, and SaaS apps, and is presented in various formats. Consolidating this data from multiple sources allows AI to organise the data and create informative insights while identifying patterns to make informed predictions and analyses. Combining data from a range of forms enhances AI algorithms as it helps to reduce biases and the limitations that occur from relying only on one set of data. Investing in data integration that supports the comprehensive collection of data to deliver real-time insights provides organisations with accurate data.
Fighting data chaos
To use data productively, it must be organised and trusted. Currently, there are some concerns about the security and privacy of data when using generative AI – it is not yet known how easy it is to hack this information and use it against the intended purpose. Due to these fears, conclusions can often be made using inaccurate or incomplete information, as humans are unsure whether they can trust generative AI with all their information. Organisations already have to comply with security measures, which will soon include legislation around AI and ChatGPT from EU lawmakers.
Poor quality or uncontrolled data can lead to organisations making ill-advised decisions, ultimately limiting their efficiency or innovation. Implementing data governance would help fight data chaos and instead lead to a sharable and automated data system.
Actionable insights
For AI products to be understood, they must be presented in a consumable and digestible manner for humans. Data is often presented in a variety of ways, such as charts, graphs and spreadsheets. While AI has the ability to create and generate data, these insights must be presented in a way that they can easily comprehend and actions are clear and understood.
Generative AI helps the data literacy divide by making it easier for organisations to create and engage with users, thereby helping them combat complex data problems. Drawing on a variety of sources and making data easier to digest and understand highlights the value of generative AI for data analytics.
Investing in the right analytics solutions can leverage the capabilities of AI and machine learning capabilities to create more powerful visualisations and dashboards to generate insights that can easily be implemented.
Connected systems
Insights from data are only as good as the actions taken from it. Connecting generative AI with operational systems can cause an automated trigger action for teams, and businesses can ultimately benefit from this transformative technology. Integrating AI-powered analytics to examine data in real time gives immediate insights and recommendations on predefined triggers or thresholds. These triggers can then be set to specific actions or alerts when conditions are met.
This is effectively a no-code automation solution which can create advanced workflows. By connecting this to all business applications, there is the potential for organisations to trigger action from their systems.
What does this mean for the future of data?
Data and analytics platforms were previously built for human use. AI will increasingly reshape how these platforms will operate in the future. A shift towards organising, consolidating and curating data for generative AI will become the norm. Ultimately creating data insights for human consumption, by sorting a huge amount of data in a more efficient and effective way.
Incorporating AI capabilities in data analytics is the way to achieve deeper insights in real time. Integrating AI into systems we already use demonstrates the human embrace of new technologies.