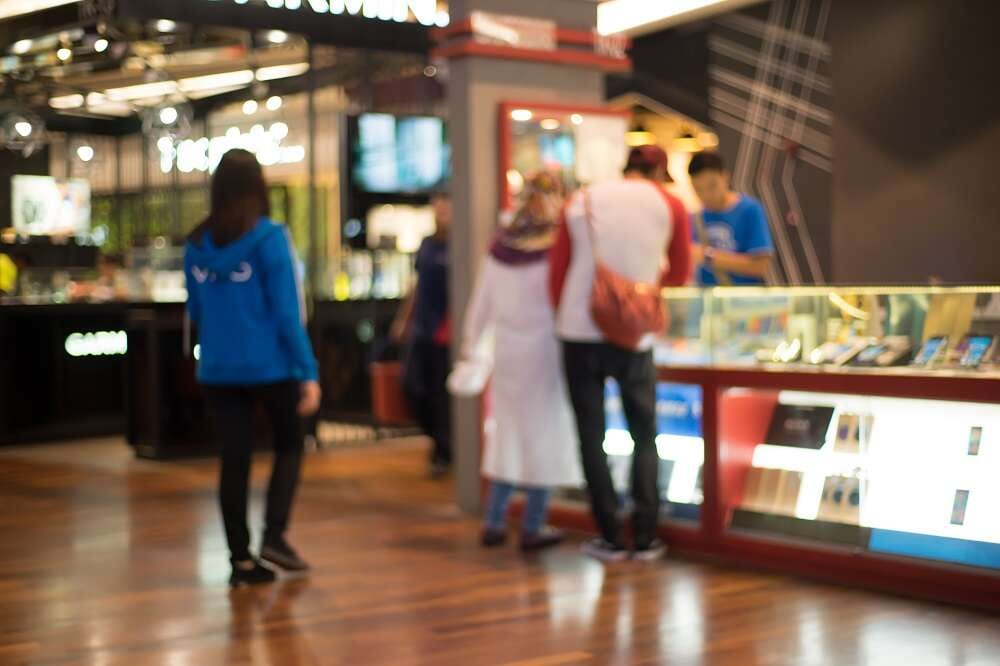
By: Chris Newbery, industry consultant Retail/CPG EMEA, Teradata
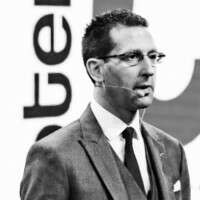
Understanding true Cost to Serve on an ongoing basis is an absolutely critical workstream to provide clarity amidst all this complexity. Yet still today, many Retailers & CPG’s can only query data at aggregated or segmented levels, meaning the picture is at best blurred, or potentially even totally inaccurate. As a result of this lack of granularity, many are missing huge opportunities to improve their margins and further enhance their customer experience.
Retailers & CPG’s are, of course, well aware of the benefits of calculating Cost to Serve. Allocating costs to specific products, channels and stores/outlets provides vital insights into which are performing well and where savings can be made. These metrics help manage the trade-offs between inventory levels, service levels and costs. However, most Cost to Serve metrics are based on assumptions, thereby making them inaccurate. No two stores are the same, and different product ranges, prices, promotions, and personalisation campaigns deliver different results in every location. Clearly no two customers are the same either, with differing preferences regarding products, experiences and channels which must always be considered.
An impossible task?
Managing data on every SKU, through every channel, for every store, and for every single customer might seem an impossible task. In many cases it is, as often data sets are incomplete, or stored in silos at department level, function level or regional level. Then, even if all the data is available, it’s all too often aggregated to make it easier to manipulate such large data sets, or assumptions are needed to make the task manageable. Humans using spreadsheets simply cannot hope to compute and action against the millions of rows of data needed to understand Cost to Serve at a customer, or individual store level.
Not for AI
This is where advanced data analytics, and specifically Machine Learning and AI, can come into their own. Enabling Retail/CPG’s of the future to deploy thousands of analytic models and provide real-time Cost to Serve by individual customer and product. These models examine millions of rows of data and spot opportunities for cost savings and/or ways to increase profitability. Only AI has the capacity to quickly and effectively analyse and decide on all the interconnected data points that are needed to deliver a comprehensive, accurate and robust calculation of Cost to Serve. As the complexity of the Retail/CPG world continues to increase, there is no better way to process the complex interactions between increasingly divers data sets. These automated data models are essential in providing managers with the precise information they need to make accurate decisions as to how to improve the performance of stores, product lines and promotions.
Don’t Guess
By its very nature, accurately calculating Cost to Serve demands an end-to-end perspective. The data must also be 100% integrated and orchestrated to support this visibility. Without this, Cost to Serve figures will be guestimates at best. To create these analytic models, data scientists therefore need access to the full range of data, whereas currently in most Retailers/CPG’s the data is often quite fragmented. Sales data can be stored in ERP systems, while stock and logistics data can be within Supply Chain management systems, personalisation data within a CRM system, and Price and Promotional data often sits within Marketing or Commercial systems. Although much can still be done to optimise processes and even automate data collection and use, if the data is in silos the ‘sum of the parts’ benefits will be lost.
Driving visibility into operations
Working with the biggest Retailers and CPG’s across the world, Teradata has helped combine accurate granular data from all parts of the business to improve and fully understand Cost to Serve. With better insights and models leveraging this data, organisations are realising both significant cost savings and revenue opportunities. For example, store-specific models that leveraged multiple data points on products, prices, promotions, and customer behaviour allowed a major US grocery Retailer to better tailor product ranges to local requirements. Ten to fifteen per cent of items in each store were changed as a result, leading to increased unit sales, margins, and overall revenue. A German Wholesaler used detailed models to calculate price point interactions at store and SKU level to predict changes in demand at specific prices. Here recommendations are now made at specific store-level, leading to 8% increase in relative net margin. In both instances, only detailed information on every aspect of the sale allowed the companies to truly understand the Cost to Serve of every product to every customer at every location.
Automating decisions
Leading Retailers/CPG’s are already deploying advanced analytic models that draw on enterprise-wide data platforms, to create far more detailed and accurate Cost to Serve insights. They are using them to make better and faster decisions, for the dual benefit of their customers and their own business. The Retailer/CPG’s of the future will take further steps to automate much of this decision-making. Analytic models and AI will predict and implement the most effective approaches to minimise Cost to Serve automatically, whilst protecting customer service and experience. They will use these enterprise data assets to re-focus and re-orientate their organisations around the customer, and then become the flexible, agile businesses they’ll need to be to continue to compete.