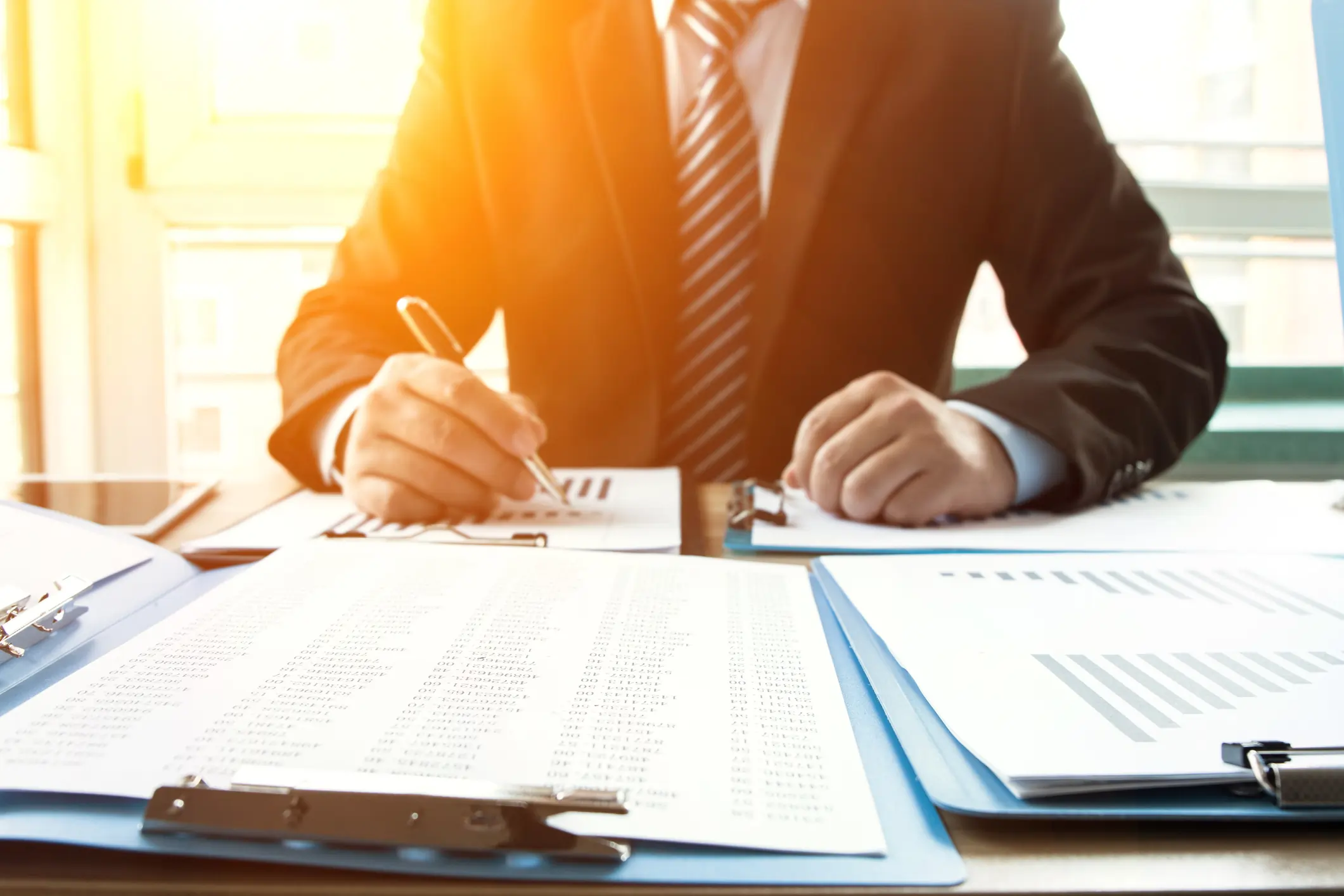
How AI Is Transforming Credit Risk Assessment
In the realm of financial services, credit risk assessment plays a crucial role in determining an individual’s or a business’s eligibility for loans and other financial products. Traditionally, this process has been heavily reliant on manual analysis and historical data, often resulting in time-consuming evaluations and sometimes inaccurate predictions. However, with the advent of artificial intelligence (AI) and machine learning (ML) technologies, the landscape of credit risk assessment is undergoing a significant transformation. This blog post delves into how AI is revolutionizing credit risk assessment, its implications, and the potential benefits it offers.
Understanding credit risk assessment:
Credit risk assessment is the process of evaluating the creditworthiness of individuals or businesses seeking financial assistance. It involves analyzing various factors such as credit history, income, debt-to-income ratio, and repayment behavior to gauge the likelihood of default on a loan or credit obligation. Traditionally, banks and financial institutions relied on manual methods and predetermined criteria to assess credit risk, often leading to subjective judgments and limited predictive accuracy.
The role of ai in credit risk assessment:
AI-powered algorithms are revolutionizing the credit risk assessment process by leveraging vast amounts of data and sophisticated analytical techniques. These algorithms can analyze diverse data sources, including transactional data, social media activity, and alternative credit data, to generate more accurate risk profiles. By incorporating machine learning models, AI systems can adapt and improve over time, enhancing their predictive capabilities and enabling more informed lending decisions.
Key advantages of ai in credit risk assessment:
Enhanced Accuracy: AI algorithms can analyze large volumes of data and identify complex patterns that may not be apparent to human analysts. This results in more accurate risk assessments and reduces the likelihood of false positives or negatives.
Improved Efficiency: AI-powered systems automate many aspects of the credit risk assessment process, significantly reducing the time and resources required for evaluation. This allows financial institutions to streamline their operations and accelerate loan approvals, enhancing customer satisfaction and competitiveness.
Risk Segmentation: AI algorithms can segment borrowers into different risk categories based on their credit profiles and behavior patterns. This allows lenders to tailor their lending strategies and pricing models to better align with the risk tolerance of each segment, optimizing portfolio performance and profitability.
Real-time Monitoring: AI systems can continuously monitor borrower activity and external factors that may impact credit risk, such as economic trends or regulatory changes. This real-time monitoring enables proactive risk management and early detection of potential defaults, reducing losses and preserving asset quality.
Inclusion and Fairness: AI-driven credit risk assessment can incorporate alternative data sources and non-traditional metrics, expanding access to credit for underserved populations. By considering a broader range of factors, AI algorithms can provide more equitable lending decisions and mitigate bias in the credit evaluation process.
Challenges and considerations:
While AI holds immense potential for transforming credit risk assessment, several challenges and considerations must be addressed to realize its full benefits:
Data Privacy and Security: AI algorithms rely on vast amounts of data, raising concerns about privacy and data security. Financial institutions must adhere to strict regulatory requirements and implement robust data protection measures to safeguard sensitive information.
Algorithmic Bias: AI models may inadvertently perpetuate or amplify biases present in the data used for training. It is essential to assess and mitigate bias in AI algorithms to ensure fair and equitable lending practices.
Interpretability and Explainability: AI-powered credit risk assessment models can be highly complex, making it challenging to interpret their decisions and explain them to stakeholders. Enhancing model interpretability is crucial for building trust and transparency in the lending process.
Regulatory Compliance: Financial institutions must navigate a complex regulatory landscape governing credit risk assessment and lending practices. AI systems must comply with regulatory requirements and industry standards to ensure legal and ethical compliance.
The future of ai in credit risk assessment:
Looking ahead, the adoption of AI in credit risk assessment is poised to accelerate, driven by advances in technology, increasing data availability, and evolving regulatory frameworks. Key trends shaping the future of AI in credit risk assessment include:
Explainable AI: As regulators and stakeholders demand greater transparency and accountability in AI-driven decisions, there is a growing focus on developing explainable AI models that provide insights into how decisions are made.
Integration with Blockchain: Blockchain technology offers potential synergies with AI in credit risk assessment by providing secure and transparent data sharing mechanisms. Integrating AI with blockchain can enhance data integrity and streamline verification processes, improving the accuracy and reliability of credit assessments.
Collaboration and Partnerships: Financial institutions are increasingly partnering with fintech startups and technology providers to leverage AI capabilities for credit risk assessment. Collaborative initiatives enable access to innovative solutions and accelerate the adoption of AI in the industry.
Ethical AI Practices: As AI algorithms exert increasing influence on critical decisions, ensuring ethical AI practices is paramount. Financial institutions must prioritize fairness, accountability, and transparency in AI-driven credit risk assessment to build trust and mitigate potential risks.
Faqs:
Q1. How does AI improve the accuracy of credit risk assessment? A1. AI algorithms can analyze large volumes of data and identify complex patterns, resulting in more accurate risk assessments compared to traditional methods.
Q2. Can AI-powered systems help mitigate bias in credit risk assessment? A2. Yes, AI models can mitigate bias by incorporating diverse data sources and implementing fairness-aware algorithms to ensure equitable lending decisions.
Q3. Are there any privacy concerns associated with AI in credit risk assessment? A3. Yes, data privacy and security are significant concerns when using AI algorithms for credit risk assessment. Financial institutions must implement robust data protection measures to safeguard sensitive information.
AI is revolutionizing credit risk assessment by leveraging advanced analytical techniques and vast amounts of data to generate more accurate and timely insights. By enhancing accuracy, efficiency, and fairness in the lending process, AI-powered systems have the potential to drive significant improvements in financial inclusion and risk management. However, addressing challenges such as algorithmic bias and data privacy is crucial to realizing the full benefits of AI in credit risk assessment. As the technology continues to evolve, financial institutions must embrace AI as a strategic tool for informed decision-making and competitive advantage in the dynamic landscape of lending and risk management.