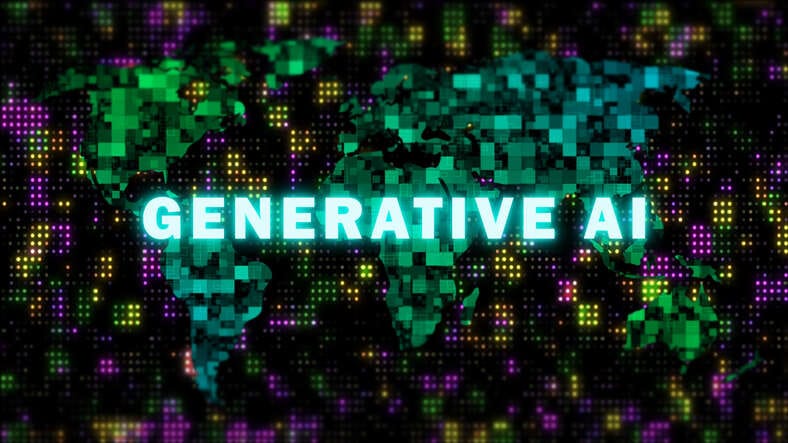
How generative AI removes the build versus buy debate
For decades, the “build versus buy” debate has remained a cornerstone of strategy discussions in boardrooms. The decision between developing custom solutions in-house or acquiring them off-the-shelf has traditionally been informed by each organisation’s unique calculation that weighs up their needs for control, customisation, and convenience against the resources available.
But when it comes to generative AI, business leaders looking to tap into the full potential of this technology are looking at this calculation and realising an alternative exists.
Rather than creating a tug-of-war between cost-effectiveness and efficiency on one side, and accuracy on the other, generative AI solutions can leverage a hybrid build and buy approach. Combining the build and buy schools of thinking will be the key to creating successful generative AI stacks that deliver impact, innovation, and ultimately, value.
The appeal of off-the-shelf is well-deserved
There is incredible innovation happening with off-the-shelf and open-sourced large language models (LLMs), however, in some cases they still fall short for organisations looking to realise the full potential of generative AI tools. Off-the-shelf, general-purpose models are incredibly smart, having been trained on vast swathes of data online, and are capable of performing a wide range of language-related tasks straight out of the box. The saved time and resources are important benefits for organisations looking to do more with less. For many businesses, this plug-and-play approach seems like the perfect solution – and one that saves on the substantial upfront costs of research and development that may be prohibitive for most organisations.
However, the convenience of the “buy” approach carries several important risks. The very same data that creates the appeal of off-the-shelf LLMs, also creates risks for organisations relying solely on pre-built generative AI solutions. For example, one drawback of this broad training data is that the model may have lower accuracy outputs, particularly when compared to the performance of a proprietary model. Luckily, while industry-specific models go some way in addressing this, fine-tuning such models further with an organisation’s own data may nonetheless involve additional costs.
The value of fine-tuned models
In contrast, advocates on the “build” side of the debate are likely to highlight the benefits of in-house solutions. Namely, their accuracy and cost in the long term, regardless of whether the solution is net-new, a proprietary model, or one that has layered unique data and governance onto an off-the-shelf foundational model. Off-the-shelf LLMs augmented with a specific dataset can help increase the model’s accuracy and business utility by combining it with external sources of knowledge. For example, augmented general-purpose models can help employees with admin like drafting personalised emails. At the same time, customisability, better accuracy, and long-term cost-effectiveness all fill in the gaps where off-the-shelf models may not perform as well.
With generative AI, these benefits ring especially true, and they clearly supplement the shortfalls of general-purpose models. An important appeal of fine-tuned generative AI models is that they are purpose-built for a specific use case, making them the most trusted alternative to off-the-shelf tools. Because these models are often trained on industry and organisation-specific data, they are less likely to produce inaccurate or made-up results – also known as hallucinations – when asked a question they may not have an answer for.
For example, a model trained on an organisation’s data will be able to understand the context of its operations and historical experiences. This can make proprietary models well placed to augment decision making, as drawing on that specialised and historic data, it can provide highly relevant and accurate outputs for organisations. For some, this could mean providing insights into how to retain an account on the risk of collapse, predict the probability of a default for a loan applicant, or even assess the viability of drug compounds in the pharmaceutical industry.
While these are important benefits, this is not to say that organisations should rely solely on “built” solutions. Not all operations within an organisation will require a bespoke AI solution, and it may be an inefficient use of resources and time to develop an in-house solution when an off-the-shelf tool can achieve the same results.
Embracing the best of both worlds
It’s evident that a one-size-fits-all solution won’t work for today’s businesses. Both the “build” and “buy” options have their benefits, which is why embracing a hybrid approach – in which companies buy a foundation model off-the-shelf and then build rules and training on top of it – will let enterprises fully tap into the generative AI advantage. Through this approach, organisations can balance the rapid innovation from the industry’s most popular tools with their need to reduce risk, boost accuracy, and stay cost-conscious.
Different models excel in different situations, and as more organisations discover this too, many will begin to combine models in similar ways.
Ultimately, the build and buy approach is not just a tactical move – but a strategic vision of where organisations should go next. It’s a shift towards a more versatile and adaptable AI ecosystem. In this landscape, businesses don’t just consume AI for efficiency gains, instead, it can be a true co-pilot in identifying opportunities for innovation and growth.