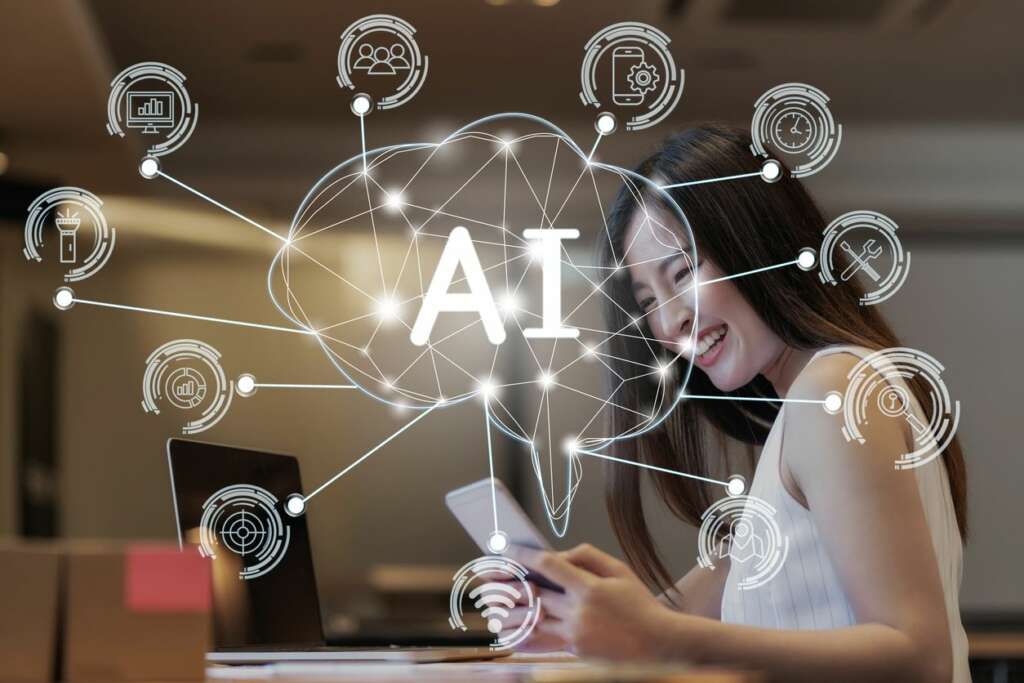
By John Andersen, Senior Director, Solution Architecture (AI/ML)
With the recent advancements in AI over the past several months (e.g., generative AI, ChatGPT), it has become abundantly clear that AI is no longer influencing back-office processes for large enterprises. Rather, it is becoming more consumer focused, empowering the lives of everyone. While this recent AI transformation and resurgence is still being researched and not yet realizing tangible business value, the time will soon come when AI is directly impacting customer experiences. What needs to happen prior to the scale that the market is predicting though? There are several key components that this paper will focus on that should be considered as high priority capabilities to fully humanize these intelligent experiences driven by AI/ML.
Personalization
A critical component to providing meaningful & intuitive experiences to individuals is around personalization. While a model may predict the same outcome to two people, the way that information should be rendered to each person could be done differently. It’s necessary then to move towards an understanding of context. This context should be derived through a combination of data & models.
There are three important attributes one should consider when looking to drive personalization: 1) Declarative Information – what data has been explicitly collected and cataloged around an individual, such as their demographic history. 2) Observations – analytics & trends that can be aggregated to observe behavioral characteristics. 3) Inferences – outputs of machine learning models to provide insights into each person, such as likelihood scores & recommendations.
Through incremental build-out of these important types of attributes around an individual, we can then begin to understand the journey that person is taking. Like how a GPS system guides you to get from point A to point B, AI should predict the trajectory that humans are taking as it relates to achieving a goal or KPI. And just how GPS systems alert you of more optimized routes based on external factors in real-time, through personalization we can alter customer journeys and impact the trajectory through more meaningful & contextual experiences. These experiences should be rendered through an omni-channel experience, sending the right contextual message, at the right time, through the right channel (e.g., web, mobile, chat).
Trust
Trust needs to be top of mind across all stages of AI, from the very beginning when considering which datasets are required to train a model, to being transparent of why a decision was made to an end user. Because trust is so critical across the development & operationalization of AI, and since the construction of an AI requires a multi-role team, then the concept of trust should extend across a variety of personas. For example, data scientists, legal/compliance, & consumers would all have very different definitions of what trust is as it relates to AI. Therefore, trust should be viewed as an end-to-end governance policy, looking at how we can enable the collaboration across teams more effectively, reducing the otherwise known friction.
When providing an insight to an end user, the first question that person might ask is, “How can I trust this decision?” This may be a difficult question to answer, especially since most models built today are still very much black-box systems. Not being able to provide a level of explanability that makes sense to a non-technical audience, may be enough for that individual to discount to insight completely. Therefore, every decision being made must have a level of explanability that can be presented to an end user. With access to a variety of methodologies, like SHAP and counterfactual explanations, models need to be transparent for consumers to leverage AI and unlock its full potential.
Another factor of trust that one should consider is around fairness/bias. Without a clear understanding of data validity and drift, it becomes difficult to understand if any bias has been introduced when training a model. This has a broader impact on risk for organizations, as well as from a regulatory perspective. Similar to how we need to impose guardrails and standards on what is deemed socially acceptable from a human standpoint, the same measurements and controls need to be applied to AI.
Finally, it is imperative that these standards & controls are imposed early on with these emerging technologies like generative AI & ChatGPT to provide the fastest path forward to unlocking business value.
Simulation
A final high-priority component to consider when influencing personal, customer experiences, is the monetary value that an organization hopes to achieve to justify the expense. While the head of a business unit is interested in the technical metrics of a model (e.g., accuracy), they also are acutely concerned with the quantifiable business value that can be realized (e.g., increase revenue), and there is a disconnect between the two.
Typically, organizations will test different types of customer experiences as it relates to a specific KPI through a series of A/B tests across a pilot population. The downside of this is that the expected target value could take a very long time to be realized. Additionally, this creates poor experiences for a subset of individuals that have been targeted with non-optimal outreaches, reducing customer satisfaction.
To replace this archaic methodology, it becomes critical to simulate the effectiveness of the outreach prior to ever deploying it in production. An airplane would never be built from scratch and tested on live passengers, rather it would run through a series of simulations to ensure it can withstand the expected factors that it would face in a reality. The same principal needs to be considered with AI, and provide the business justification that the value will be achieved in a reasonable timeframe.
Conclusion
Through the recent transformation of AI, and the shift from back-office processes to consumer based, AI will unlock new avenues never previously thought of. To provide a more seamless transition & adoption, however, we must look across these three critical pillars of personalization, trust, and simulation to take full advantage of what AI is now possible of.