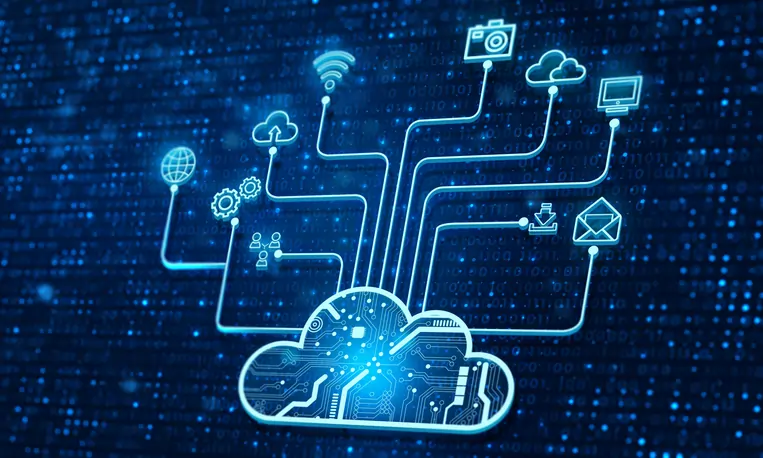
Implementing AI? What Lessons Can Be Learnt From The Cloud Era?

When looking at the potential growth opportunities for AI, there is one obvious place to draw a comparison: cloud computing. The cloud has been one of the modern technology industry’s outstanding success stories. In its contemporary form, the market is still in its teens, with the likes of AWS, the Google Cloud Platform, and Microsoft Azure all appearing in the mid to late 2000s.
These public cloud services added huge momentum to the wider market, and by 2018 the industry as a whole was worth over $150 billion worldwide. Fast forward to today, and the market is expected to grow from over $675 billion this year to an eye-watering £2.3 trillion by 2032.
As impressive as this clearly is, AI looks likely to deliver significantly faster growth. There are a range of estimates out there, with one putting the sector’s value at $621 billion this year – that’s less than two years since ChatGPT launched. For anyone of the opinion that this is more hype than substance, it’s worth noting that Accenture alone booked over $600 million in generative AI business for the second quarter fiscal 2024, putting them on track for up to $2.4 billion annualised.
This, however, turns out to be a comparatively small sector. By 2032, the AI market could overtake the cloud industry, hitting over $2.7 trillion in value. Look elsewhere, and the numbers become truly mind-boggling, with one research organisation estimating it will top $30 trillion.
The benefit of hindsight
With all this in mind, are there any lessons we can take from our collective experience with cloud adoption that might prove useful for nascent AI strategies? There are certainly some familiar threads beginning to emerge, with the fear of missing out once again playing a major role.
Back in the earlier days of cloud adoption, excitement about the technology saw organisations racing to outsource their infrastructure and applications without full consideration and planning. For example, it’s now clear that many lacked a clear understanding of which types of cloud services were best suited to different types of data. The result was that the choice of cloud infrastructure model and service provider was often mishandled and had to be rectified later on, with the additional expense and inconvenience that generally brings.
Similarly, deployment teams could quite easily underestimate the inherent complexity of cloud migration processes, leading to prolonged system downtime and, in some cases, data loss. Moreover, when data volumes began to increase, a lack of effective scalability brought with it almost inevitable performance issues. Looking back now the list of challenges was lengthy, with security, risk management, and compliance among the other hurdles facing IT teams as they went looking for the benefits cloud clearly had to offer.
Herein lies an important point: applying this kind of hindsight to the development and deployment of AI technologies can help organisations avoid similar mistakes this time around. Comprehensive planning is particularly important because adopting exciting new technology is only one piece of the jigsaw. To deliver on ambitious business objectives, the process also requires effective transformation strategies and a systematic approach to technology implementation.
In practical terms, effective AI adoption needs a solid foundation based on a clear understanding of organisational objectives. Is AI being considered to address efficiency or profitability? Is it about innovation and competitiveness, or is it being driven by the concern that rivals are going to bring something disruptive to the market? Creating solid answers to questions like these is key to getting off on the right foot.
Part of the problem is that almost everyone is learning how to implement AI at the same time. There is also a dearth of talent and experience in the market, which, when added to the kind of demand being seen by the likes of Accenture, presents some obvious barriers to understanding what best practice looks like. In this context, leaders need to exercise caution when evaluating which partners to work with.
The devil’s in the data
While these issues are clearly an important part of the picture, what almost everyone will have in common is the pivotal role data will play in whether their AI efforts are successful. AI systems are only as effective as the data used to build their models, and it makes sense that inaccurate, incomplete or poorly maintained data sources are likely to cause major problems.
For example, many organisations today rely on a wide variety of complex and disparate datasets that, for AI purposes, may need to be brought together from different cloud platforms and siloes. This can easily become a very complex process, and organisational leaders should be cautious about rolling out major AI projects without first putting a well-defined data management strategy in place.
On the other side of the equation, ensuring accurate, reliable AI outputs is an ongoing, holistic process. Even if data sources have been selected with the utmost care, the performance of AI systems should be constantly monitored to ensure that algorithms remain fit for purpose.
The same goes for security and data protection. The risks associated with AI misuse are already making headlines, while at the same time, governments and regulators are rolling out new rules to enforce compliance. In March, for example, the EU’s new AI Act came into force, which, according to the policy announcement, is “part of a wider package of policy measures to support the development of trustworthy AI” that will “guarantee the safety and fundamental rights of people and businesses when it comes to AI.”
Put all of these factors together, and it’s clear that delivering on the potential of AI requires much more than picking processes to automate or tools that will ‘revolutionise’ the way organisations operate. The opportunities are certainly out there, but in the years ahead, success will most likely depend on a measured approach that balances urgency with careful planning and implementation.