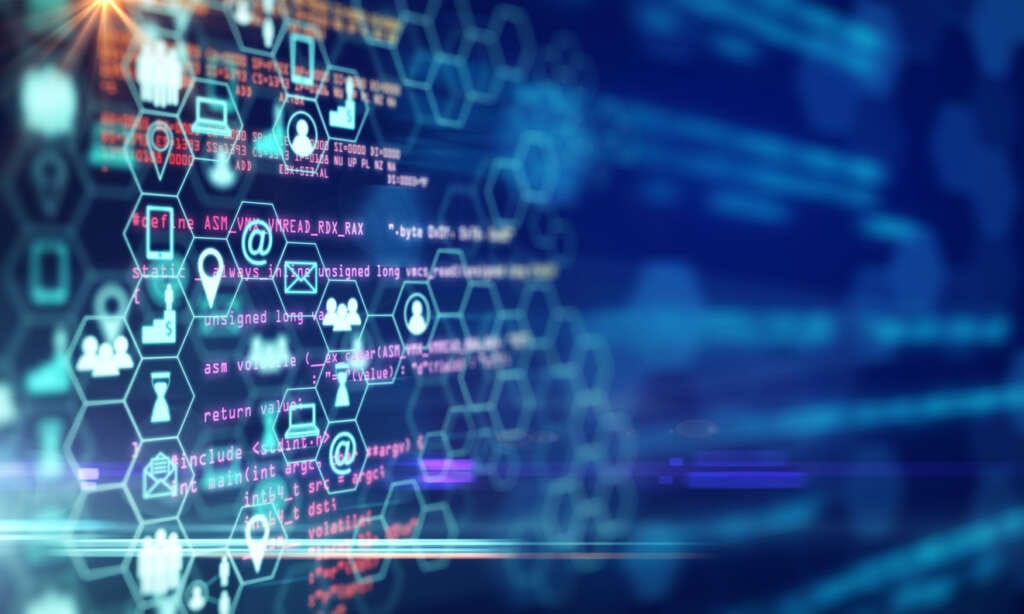
Large language models: how boards should approach generative AI
Mike Drew, partner and head of the technology & IT services practice, looks at the business risks posed by implementing generative AI, the precautions boards can take in the absence of regulation, and how they can do it safely
In April of this year, Samsung Electronics implemented a ban on the use of ChatGPT and other AI-powered chatbots by its employees. The decision came after the company initially employed ChatGPT to assist their engineers in resolving source code issues. This resulted in a significant data breach with sensitive information, including the source code for a new programme, internal meeting notes, and hardware-related data, being leaked to the public.
Samsung is not alone in taking action against the use of ChatGPT and similar tools by employees. In January, Amazon issued a similar warning to its staff, instructing them not to share any code or confidential company information with ChatGPT. The decision came after Amazon reportedly discovered examples of ChatGPT responses that resembled internal Amazon data.
Following suit in February, JPMorgan Chase imposed strict limitations on the use of ChatGPT by its employees due to concerns about potential regulatory risks associated with sharing sensitive financial information. Subsequently, other major U.S. banks, including Bank of America, Citigroup, Deutsche Bank, Wells Fargo, and Goldman Sachs, also adopted similar measures.
Boards who aim to utilise tools like ChatGPT or develop their own large language models (LLMs) face an additional hurdle in the form of biases. ChatGPT, in particular, has been found to reflect political and cultural sentiments rather than providing impartial analysis. Moreover, companies employing AI algorithms, for example hiring algorithms, frequently encounter biases due to the datasets on which these algorithms are trained, which can incorporate historical racist and sexist data.
Amazon, for example, had to abandon an experimental AI hiring tool after it autonomously learned to favour male candidates. The AI system exhibited a bias by penalising CV’s containing words associated with women and even downgrading candidates who graduated from all-women’s colleges.
Yet despite the risk of data leaks, breaching regulatory laws, and ingrained biases, LLMs like ChatGPT can enhance customer experiences, reduce costs, and increase productivity. It’s understandable then that boards would want to implement the technology in their organisations. But how can they do this safely, particularly in the absence of regulation?
License LLMs
Implementing technical measures and licensing an LLM can be an effective strategy. By licensing the software, boards can establish enforceable legal agreements that dictate how their data is handled and where it is permitted or prohibited from being shared. Licensing LLMs enables the protection of confidential information, sets regulations for data storage, and provides guidelines on how employees can utilise the software.
Develop an ‘in-house GPT’
Another option available to boards is the development of their own GPT or engaging companies to create a customised version. By creating an ‘in-house GPT’, boards can ensure the software contains only the specific information that employees are authorised to access. Furthermore, boards can take measures to safeguard the information fed into the system, whether they develop it themselves or collaborate with an AI company to create a secure platform for data input and storage.
Establish data governance and responsible AI guidelines
Boards can adopt a responsible AI initiative, which revolves around a set of principles emphasising accountability, transparency, privacy and security, as well as fairness and inclusiveness in algorithm development and deployment. This proactive approach also prepares companies for potential future AI regulations and promotes responsible and ethical AI practices. Aligned with this is the establishment of data governance practices, ensuring the quality, accuracy, and fairness of the training data by thorough review and cleaning to minimise biases and problematic patterns.
Introduce bias detection
Alongside the development of an in-house GTP or licenced model, boards should implement processes to detect and address biases within the generative AI system. This can be done by regularly evaluating the model’s outputs and analyse for any potential biases based on various criteria such as gender, race, or cultural background. Bias mitigation techniques, such as de-biasing algorithms or diversifying the training data, can be applied to reduce biased outputs.
Appoint senior leaders with expertise in AI
Senior leaders well-versed in AI and its associated risks can contribute to strategic decision-making when implementing LLMs. CTOs and CIOs can provide insights on potential risks, recommend appropriate safeguards, and guide the organisation’s overall AI strategy, while also working toward developing an ethical framework specifically tailored to the organisation. They can collaborate with cross-functional teams to establish guidelines and policies that prioritise fairness, accountability, transparency, and privacy in AI implementation. What’s more, they can monitor potential regulatory developments, ensure the organisation’s AI practices align with potential future laws, and proactively implement necessary measures to adhere to ethical standards.
For boards, taking a proactive approach to ChatGPT implementation risk serves two purposes. It mitigates the very real threats the technology poses to organisations. It also prepares these organisations for the regulation inevitably on the horizon. While boards face a Wild West in the current generative AI landscape, this will not always be the case; having guidelines and policies in place means organisations can adapt quickly, and gain competitive advantages, when AI laws focusing on generative AI come into effect.