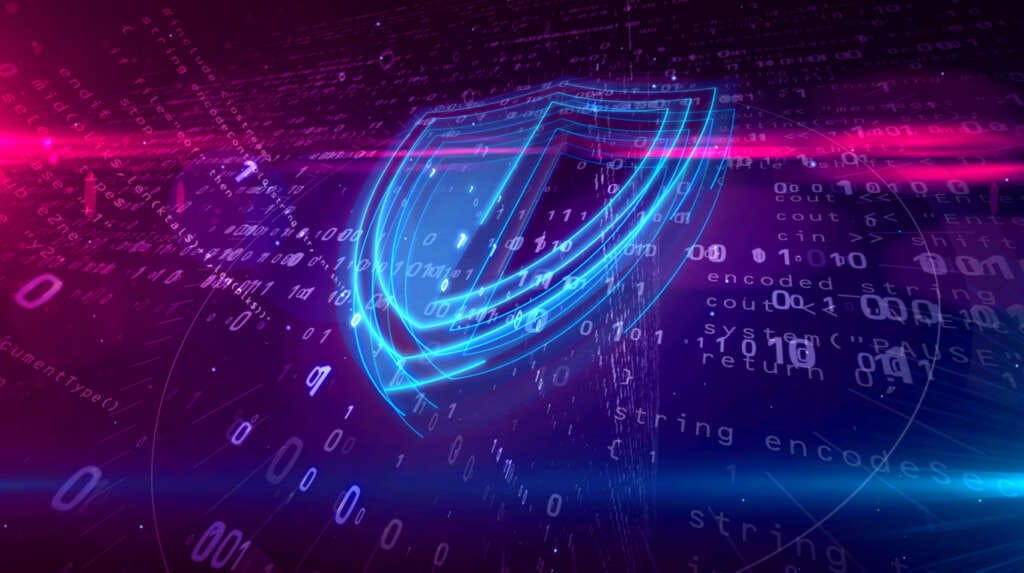
By Matthew Negus, Sam Lowe, Libero Marconi in the Disputes & Investigations team at A&M
The rapid adoption of new technologies has shifted the global economy into a period of unprecedented change which the World Economic Forum has labelled the Fourth Industrial Revolution.
Artificial Intelligence (AI) is key in driving this phenomenon, with potential benefits in productivity, decision-making, automation of tasks and efficiency across all industries. AI is also seen as an important source of future value, with OECD figures tracking a five-fold increase in venture capital investment in this area from 2016 to 2021, to over $200 billion.
Against this backdrop, adoption of AI is often an imperative for businesses’ digital transformation initiatives, with projects across core business operations, customer-facing services and corporate functions.
Within financial services, for example, organisations are investing in AI tools, with use cases emerging across a range of areas, including fraud detection, transaction monitoring, conversational AI, algorithmic trading and underwriting activities.
However, while AI systems offer many benefits to businesses and consumers, their scale, complexity, and use for tasks which can be sensitive in nature, mean they also present challenges with significant potential risks to organisations, individuals, and wider society, including discrimination and harm to vulnerable individuals.
National governments, regulators, NGOs, and industry, while recognising the opportunities, have also acknowledged the need to address these potential harms.
This can be seen in initiatives such as the White House publication of a Blueprint for an AI Bill of Rights, the National Institute of Standards and Technology (NIST) AI Risk Framework, the work of the Centre for Data Ethics and Innovation in the UK, and public statements by organisations on their adoption of AI principles as part of AI ethics or Responsible AI movements.
There is also an expanding raft of laws and regulatory proposals addressing AI use. This includes the draft EU AI Act and AI-related requirements in the EU Digital Services Package, a New York law on the use of AI for hiring decisions, a Colorado law prohibiting algorithmic bias in insurance decisions, and various sectoral developments in rules and standards.
Data privacy and cybersecurity are often central considerations in relation to these concerns and subsequent rulemaking. For organisations to adopt AI successfully, managing risk in these areas will be essential. Addressing such risks begins with identifying where they may arise.
AI and Data Privacy
The most obvious point is that, in so far as AI systems and projects make use of personal information subject to data protection and privacy laws, these rules will apply to the use of AI, particularly where this personal information is used as part of the training data sets for machine learning. The development, procurement and deployment of AI should therefore fall within existing privacy programs and processes.
While requirements vary across jurisdictions, organisations wishing to establish a strong foundation for privacy-respecting use of AI should follow principles of privacy by design, transparency and individual choice and control.
For AI use in scope of the EU General Data Protection Regulation (GDPR), other prominent considerations will be the lawful basis for the use of personal information, performance of data protection impact assessments where there is a high risk to individuals, and appropriate data management practices, such as accuracy, relevance and retention.
Finally, the GDPR prohibits fully automated decision-making with a significant impact on individuals, such as determinations of creditworthiness, so this will need to be subject to compensating controls to ensure there is some degree of manual review taking place.
Alongside requirements deriving from regulation, privacy is often viewed as a core principle of ethical or responsible AI. Irrespective of applicable privacy laws, organisations wishing to adopt or demonstrate their adherence to these principles should make sure they have robust AI privacy practices. This will be particularly important where these statements are public to avoid potential reputational or regulatory risks.
There are also considerations that warrant particular attention due to the nature of AI systems. The size of datasets required to develop and train AI models presents myriad privacy issues that can arise during the collection or acquisition, use and ongoing management of data. There are trade-offs between minimising data and making sure AI models are accurate, and tensions between checking for bias while avoiding collecting more sensitive data categories such as ethic origin or sexual orientation.
Finally, where AI projects involve processing of personal information or decisions which may adversely affect individuals, there may need to be consideration of whether AI use is appropriate at all, considering the aims and context of the activity.
Privacy professionals, with their experience in making judgments on principle-based issues affecting individuals across the full range of organisations’ activities, are often well-placed to support the nuanced, ethically based decisions that accompany defensible AI adoption.
AI and Cybersecurity
Alongside privacy, cybersecurity presents many challenges for AI implementation. The World Economic Forum’s Global Risks Report 2021 considers cybersecurity failure among the greatest challenges confronting the world in the next decade. AI systems present significant security risks due to their complexity and are often targets for hackers. As AI becomes increasingly embedded across organisations, fresh questions arise about how to safeguard systems, and their underlying algorithms, against attacks.
Organisations typically do not have one standard they can follow which addresses all cybersecurity issues, and it therefore requires consideration of AI security risks across a range of areas. To address the vulnerabilities of AI, engineers and developers, working alongside cyber specialists, need to evaluate existing security methods, develop new tools and strategies, and formulate technical guidelines and standards.
As noted above, AI development relies on the availability of large amounts of data to train algorithms which brings corresponding security risks. The more data generated and the more users with access, the higher the chances of data leakage and subsequent misuse. A key consideration for organisations will be identifying the best data management environment for sensitive data and training algorithms.
AI also presents new vulnerabilities. So-called “connectionist AI” systems that support safety-critical applications such as self-driving vehicles, despite achieving “superhuman” performance in complex tasks such as manoeuvring a vehicle, can still make critical errors based on misunderstood inputs.
Organisations will need to manage the trade-offs between the expense of high-quality data required to train large neural networks and security risks. The alternatives of procuring existing datasets and pre-trained models brings the potential to introduce unknown issues.
Threats also arise from attacks that introduce malicious data or meaningless noise to AI systems, causing them to make errors in their intended tasks, such as causing autonomous vehicles to misinterpret Stop signs. These issues are further complicated by the often “black box” nature of AI systems which can make it extremely hard to clarify why or how a result was arrived at.
The first line of defence is to prevent attackers from gaining access to AI systems. However, neural networks are transferable, which allows attackers to train systems on replacement models that teach malicious examples, even if the data is labelled correctly. Defining representative datasets to detect and combat malicious examples can be difficult.
What should organisations do?
Issues with data privacy and cybersecurity represent significant risk factors for organisations’ AI activities, with the potential for reputational damage, impact on consumer trust, regulatory scrutiny and large fines, legal claims, or the delay, derailment, or shutdown of initiatives.
Conversely, strong data privacy and cybersecurity stances can be important enablers for AI adoption and the commercialisation of this technology. Organisations should develop a strategy for how they will manage these topics, calibrating responses to their AI commercial goals, use cases, risk appetite and values, and industry standards.
At an organisational level, there should be clear governance for AI projects addressing oversight and requirements for data privacy and cybersecurity. This includes representation of AI within wider technology or risk management committees or working groups and policy requirements, with clear escalation processes for high-risk issues, and strong working relationships between control functions and business areas.
Organisations should also adopt or develop AI risk frameworks and embed relevant privacy and security controls at appropriate stages of AI development and procurement lifecycles. This should be complemented by certification of data and AI-training processes, continuous assessment, application of decision logic, and standardisation.
AI sandboxes, either within organisations or in cooperation with regulators, may also offer a helpful means to manage risks in a safe, isolated environment during the development stages of AI systems.
There should also be consideration of training and awareness needs, and making sure relevant teams have the appropriate skills and expertise to identify, assess and remediate issues, such as product development and technology teams.
Organisations contemplating more widespread or higher-risk AI usage activities may also want to explore the use of privacy-enhancing technologies, security technologies, and AI assurance tools in support of data privacy and cybersecurity objectives.
Finally, horizon scanning for regulatory developments and sectoral standards, and regularly engaging with peers, industry groups and external experts on these issues can help to identify upcoming challenges and maintain activities consistent with accepted good practices.