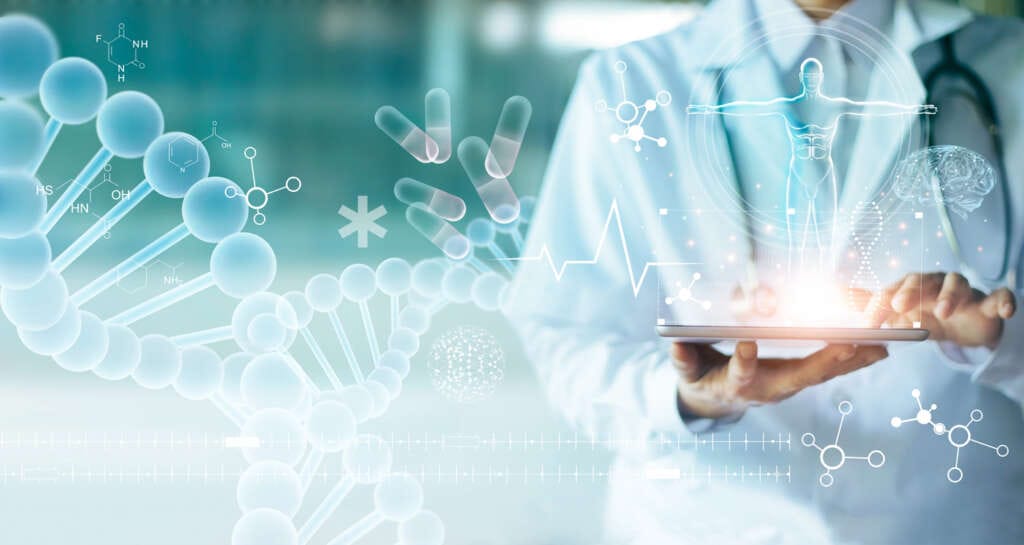
By Alessandro Raimondo, Medical Applications in the Knowledge Transfer Group at CERN
It’s no secret that collaboration and knowledge sharing is how the world can improve. They are also a fundamental part of any scientific research project. At CERN, we developed the World Wide Web so that scientists could share their data with other researchers across the world. We push the limits of science and engineering, and partner with industry to enable innovation with positive societal impact. Collaboration and knowledge sharing are vital in healthcare, where hospitals are digitalising their records, creating opportunities for improved methods for diagnosis and treatment.
At CERN, our mission is to perform world-class research to uncover how the universe works. We do this by providing a unique range of particle accelerator facilities to researchers, including the Large Hadron Collider, the world’s most powerful particle accelerator stretching 27 kilometres 100 metres underground. In many ways, the human body is much like the infrastructure we use at CERN to understand the extremes of our universe. The LHC is complex, sophisticated, runs in some extreme conditions and as such, has many systems running at once. It produces large volumes of raw data, which is heterogenous and needs to be cleaned and prepared before analysis. To ensure that each part of this system is working and maintained, we rely on machine learning (a subset of artificial intelligence) that can help us to predict and diagnose anomalies to keep our infrastructure working around the clock. Just like anomalies need to be predicted well ahead of time to maintain a healthy body.
At CERN’s Knowledge Transfer group, we bridge the gap between science and innovation by finding unique ways to apply our specialised knowledge beyond particle physics. Just as we adapted our tools for CERN’s challenges, we can draw on our AI expertise for medical applications by working in close collaboration with our partners.
For example, CERN scientists have adapted algorithms used to detect anomalies in the welds of the Large Hadron Collider to detect anomalies in the body from medical scans. Using AI, the algorithms analyse, predict and classify pathologies in the brain from MRI scans. Another algorithm used to prevent failures in our complex systems could be used to predict the probability of a patient developing breast cancer. Both these medical contexts are completely different to the intended use of the algorithms, but they are similar in their complexity. What is central is the opportunity to collaborate so that the capabilities unlocked in research at CERN can be harnessed for good in medical contexts.
Improving AI models while keeping patient data confidential
Although AI techniques from scientific research can be translated to healthcare, one difficulty and limitation is patient data privacy. This is particularly important as AI performs best on large and diverse datasets. While data privacy is generally not an issue in a physics research context, in the medical world, it is one of the biggest ethical and legal considerations. To take advantage of the benefits of sharing knowledge and working collaboratively in a medical setting, we needed a new approach to train our AI while ensuring data privacy.
We decided on federated learning, a machine learning method where algorithms are trained across multiple decentralised servers that each hold data locally. In partnership with medical institutions across Europe, CERN experts applied this concept to the medical domain, developing a secure way of sharing information while ensuring the confidentiality outside of each hospital or medical facility. This involved the creation of separate installations that could each be hosted within the hospitals themselves, where the algorithms are locally trained on each set of data.
Without exchanging any data, the resulting platform works by sharing each hospital’s algorithm and parameters considered (representing the knowledge acquired from the data). This method allows clinicians to analyse, diagnose and prognose diseases with machine learning while crucially keeping patient data confidential
The collaboration between different disciplines for this project has been essential for the success of the developed applications. It allows not only the application of AI from particle physics research to healthcare, but also creates opportunities for cross-fertilisation of knowledge and methodology between two distant fields. This complementary approach is vital to ensure the successful application of AI within healthcare, allowing for its full potential to be reached.
Through innovative partnerships like these, CERN can share its expertise, knowledge, skills and capabilities from the extremes of science to accelerate positive change in society. Whether it is in the medical field, or beyond, time is ripe to work with unique research organisations.