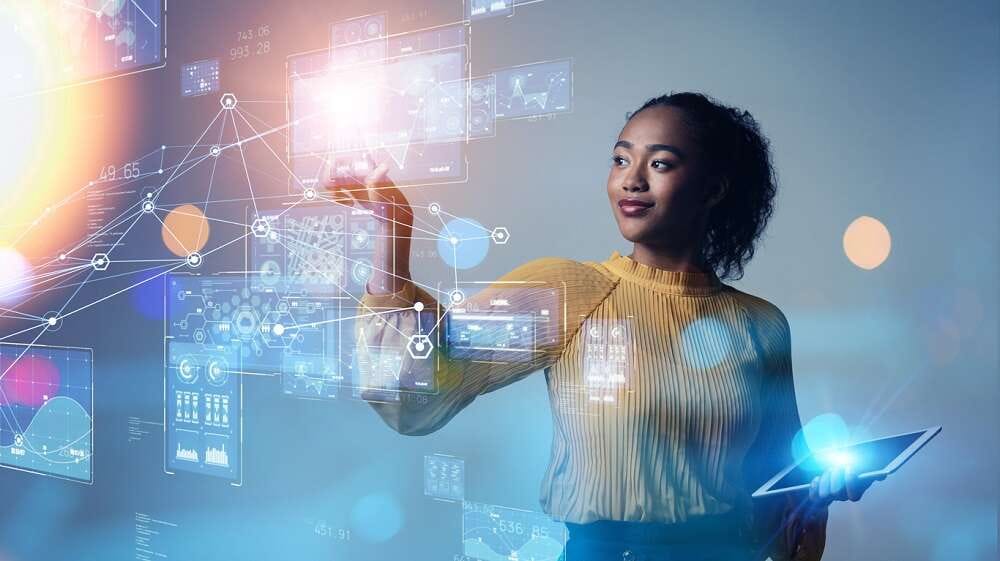
By Gareth Brown, Clir Renewables
The world needs clean power. The latest report by the Intergovernmental Panel on Climate Change (IPCC) has made that perfectly clear if we are to cap global temperatures at 1.5 degrees Celsius.
At the Conference of the Parties 26 (COP26) summit, it was suggested that developed governments need to mobilize $100 billion in public climate investment each year, with much of that headed toward renewable energy projects. Despite this investment, unlocking much more private investment is clearly needed to fund renewable energy projects and reach net zero.
The International Energy Agency suggests that the world needs to unlock further private investment for clean energy to curb climate change and that the current level needs to triple. That’s why data is needed to reduce risk for private companies that are making environmentally responsible investments.
The central challenge for renewable energy investors is risk, driven in part by the intermittency problem. It’s not always sunny and it’s not always windy, so power generation and revenue is largely influenced by the weather – unlike fossil fuels which can be burned on demand.
Using the latest advances in data, including artificial intelligence (AI) and machine learning (ML), we can increase the availability of renewable energy production, drive higher revenue and mitigate performance issues. With investors seeking more stable and predictable returns, these methods significantly reduce financial risk, unlocking the potential for further investment in this crucial sector.
One of the main ways that the sector can use data to reduce risk for investors is by optimizing energy production and managing underperformance. This can help forecast more accurate production budgets and returns on investment. By collecting data on asset performance, owners have precise insights into which turbine or solar panel is underperforming.
For example, if a wind farm is not producing its anticipated energy yield, software can flag underperformance, allowing technicians and asset operators to investigate a variety of potential causes, including wind strength, direction, wake effects or mechanical issues within the turbine. Moreover, data can inform wind farm operators of the most strategic times to conduct planned maintenance. Clearly, this shouldn’t happen during the windiest period of the year, where much of the revenue will be generated.
Data can also inform asset owners of project performance. Instead of relying on an operator or asset manager for static reports on performance and wind resource against budgets, data allows for increased visibility into the project. Constant visibility into reporting, yield analysis and financial budgeting allows investors clear oversight into the performance of their asset. The data allows investors to benchmark the reports from the operator and ensure that their investment is generating as much return as possible.
Underperformance of assets is a common issue in the renewable energy industry. As there are many original equipment manufacturers (OEMs), it can be difficult for operators to have a complete understanding of how components perform in different scenarios. With access to an industry-wide dataset and a deep understanding of the common faults of each product, operators can learn from other projects across the world that use the same machines.
For example, if a turbine has a fault during low temperatures in the winter, this data can be collected and used to provide insights into the performance of another wind farm using the same turbines. The operator can act accordingly to keep the machine up and running most efficiently, knowing that this piece of equipment struggles under those circumstances. The more data that is collected, the more effective asset operators can be at increasing and maintaining the production of energy at a wind farm, reducing the amount of down time.
To maximize the benefits of data in this way, you need extremely large datasets and analysis on gigawatts (GW) of operational data. It can be a challenge to collect the necessary amount of data, especially as asset owners or OEMs are often reluctant to share the performance of their projects with competitors. Anonymizing this data is essential for it to be shared and have maximum impact.
Data is central to reducing financial risk to investors in renewable energy projects, and can be used to manage performance and underperformance, reduce downtime and increase energy production. This is essential not only to the investors on a specific project, who can stabilize the risk on their investments by having greater oversight into the performance of their asset, but to the industry as a whole.
By mitigating down time and being able to better predict energy production, investors are more inclined to invest in renewables as the risks are lower. If we are to reach the ambitious climate targets outlined during COP26 and successfully unlock vast amounts of climate capital, it’s essential that the renewable energy sector uses data to provide clarity, improve performance and lower risk for the private investors that will be central to the energy transition.